· Insights · 10 min read
AI Without Compliance: A Cautionary Tale of FTC Enforcement
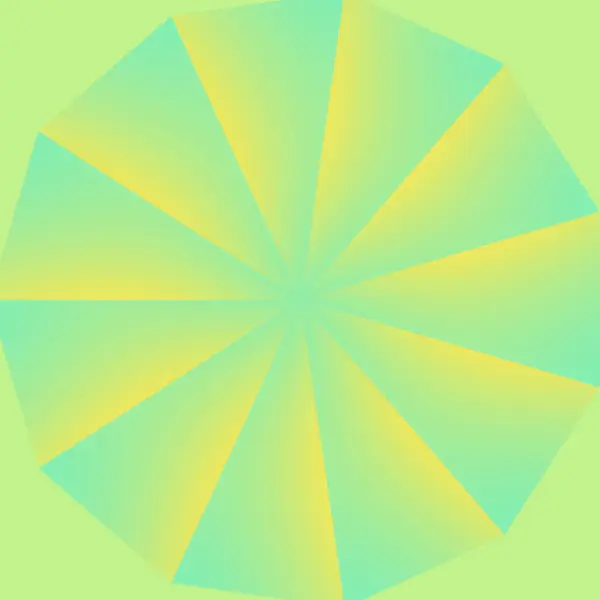
The FTC is intensifying scrutiny of AI and data companies, focusing on unfair or deceptive practices in data collection and use. Recent enforcement actions include substantial penalties and “algorithmic disgorgement,” requiring companies to delete AI models trained on improperly obtained data. Companies must ensure robust compliance strategies, particularly in data acquisition and AI model development, to mitigate significant financial and operational risks.
First, software was eating the world; now, it’s supposedly AI – or the data used to create that AI – that’s eating the world. Either way, the market for products and services powered by machine learning and artificial intelligence is hot, with even “traditional” companies and industries realizing the benefits of these technologies.
Ever the trend-follower, the FTC has also taken notice of these areas (cue the dramatic music). In the past two years, they have increased their focus on both AI companies specifically and data companies generically. While it’s clear that the FTC isn’t diving deep into the architecture of transformer models or MLOps platforms, they are developing a high-level understanding of how companies acquire data and use this data to train models variously described as machine learning, deep learning, NLP, or AI.
As the FTC’s attention and insight have grown, they’ve become more aware of how issues with data collection and acquisition interact with their jurisdiction – especially as it relates to privacy and consumers’ rights. Today’s post will cover some recent developments in FTC oversight and enforcement, as well as how organizations can avoid, identify, and mitigate the risks related to “tainted” models.
A Cautionary Tale for Investors
Picture this: You’ve just invested in a company that uses machine learning to provide the coolest [✨INSERT FAVORITE DISRUPTIVE TECH✨] to its customers. Things are looking up - the market is hot, and customer growth is accelerating. But what happens when the FTC issues a complaint against the company alleging that their data collection or retention practices are illegal?
Well, as it turns out, not only is the company potentially liable for a fine, but they may also be forced to delete all of the illegal data AND ANY ALGORITHMS DERIVED FROM OR TRAINED ON the data. Let that sink in for a minute. All of the derived models are gone.
What impact is this going to have on the company? Are they still a going concern? Are you going to have to write off this investment?
These aren’t just fictional campfire tales to scare tech investors. They’re real-world nightmares that companies are facing today.
Federal Trade Commission
Oversight
The FTC is responsible for enforcing over 70 different laws, but they’re best known for their purview over unfair or deceptive practices. When applied to the area of machine learning, they generally focus on whether companies are utilizing consumer data in unfair or deceptive ways. Oftentimes, this means that the FTC investigates whether companies violated federal regulations related to the collection and use of consumer data.
There are multiple federal regulations that govern the collection and use of consumer data: the Children’s Online Privacy Protection Act (COPPA), for example, requires notice and verifiable parental consent prior to the collection, use, or distribution of children’s data. The FTC is able to directly fine organizations for failure to comply with COPPA; in addition, they can work with the courts to impose additional penalties.
B2C Only? Or B2B Too?
Many companies in the B2B space have historically ignored the FTC and its Bureau of Consumer Protection; if the FTC does cross their radar, it’s typically because the Bureau of Competition is involved, like in the case of a Hart-Scott-Rodino (HSR) review prior to closing a business combination.
Increasingly, however, the FTC and some courts have begun to apply “consumer protection” concepts to B2B relationships. In the context of machine learning, these causes of action are often supplemental to traditional breach of contract claims like confidentiality and purpose of use; that said, they do evidence an increasing risk for companies that “push the boundaries” of their data acquisition strategies. For B2B companies doing business in the UK and EU, the headwinds blow even stronger.
Increased Scrutiny and Settlements
When it comes to consumer data, the FTC has clearly been stepping up its focus on illegal collection. But in many cases, companies didn’t stop at collection; they went on to use that illegally-collected data for other purposes. Sometimes, those purposes were themselves illegal (i.e., “illegal use”); in other cases, while the purpose of use was not prohibited, the initial collection or other practices arguably tainted “downstream” IP.
One recent example of this latter category occurred in Weight Watchers/Kurbo’s (now just “WW”) settlement with the FTC. While there was nothing inherently illegal about a weight loss application using machine learning to customize programs, they violated the COPPA when they failed to properly obtain parental consent for the collection of children’s data.
ROI: Regret on Investment
Kurbo was acquired by WW in 2019 for $3M. As part of the FTC settlement, WW will pay a $1.5 million civil penalty and delete all of the illegally collected data. While we don’t know what the reps and warranties in Kurbo’s purchase agreement were, we can at least agree that erasing 50% of deal value isn’t the return that WW was hoping for.
Some companies view fines with a risk-based approach: how do the penalties compare to the potential revenue generated by the wrongdoing? This approach may no longer be viable, as the FTC seems to be catching on to this incentive dilemma, and they’ve got a new tool in their enforcement arsenal.
Algorithmic Disgorgement
Simply put, the FTC needed to change the “expected value” of the non-compliance strategy, as companies continued to skirt the rules, knowing that even if they were caught, it was still an “NPV-positive” decision. Penalties, while a disincentive, weren’t enough to outweigh the value of the IP created.
So, what’s the easiest way to change that? Take away the IP. Blow it up. Put it in the trash. Make them watch it burn.
The FTC’s attorneys are a little more serious than we are though, so they picked a technical term – disgorgement. Disgorgement is often used as an equitable remedy to prevent unjust enrichment: it most typically requires a party who profits from an illegal act to give up any profits arising out of that act.
In the case of algorithmic disgorgement, the FTC went back to its original meaning under Black’s Law Dictionary – “the act of giving up something.” In essence, algorithmic disgorgement requires the offending party to delete or destroy any algorithms, models, or other work product or IP derived from or trained on ill-gotten data.
While the FTC has only required algorithmic disgorgement twice previously, both of these have occurred in the past year. In 2021, the FTC required Everalbum to delete the facial recognition models and algorithms that had been built using customer data after they failed to properly obtain consent.
These two cases serve as a warning to organizations: building and training models on tainted data opens the door to serious FTC actions. The frequency of settlements involving algorithmic disgorgement are almost certainly going to increase in the near future.
Why Does this Matter?
Companies often spend months to years building machine learning models and launching them into production. But if the rights to collect and use the training data weren’t secured or documented, what happens to that investment?
The FTC has now demonstrated that such investments can become complete write-offs in the blink of an eye.
The impact of tainted data flows through an organization.
Writing off valuable IP assets and removing product functionality is likely to leave an even bigger mark on the operational and strategic picture; the operational efficiency of many companies today is based largely on their technology-enablement through machine learning. What happens to their labor costs and valuations when that technology is disabled?
For many single-product or API companies, these risks are existential.
Avoiding the FTC’s Naughty List
For Companies and Founders
If you’re building machine learning models, start with the data. Where did it come from? Does your organization have someone you can talk to about your contracts or applicable laws? Just like good code, documenting your findings and decisions at this stage is critical.
For organizations that create many machine learning models or frequently iterate and enhance on key models, information systems dedicated to tracking your training data might be good investments. Luckily, many MLOps platforms can track the provenance and metadata that supports this compliance. But, as always, it’s a garbage-in, garbage-out situation. If the systems of record upstream from your training data don’t collect critical information like consent, then there’s no way to track this downstream in your training artifacts.
Strong data protection policies and related procedures also help ensure that no tainted data ever enters a training set or leaks to an unauthorized party. If you do discover that models essential to your operations contain problematic data, redevelopment of the models is often the best solution.
For Investors or Acquirers
If you’re investing in or acquiring a company whose value is predicated on their models or ML/AI/data science capabilities, then it’s essential to do proper technology due diligence. Sometimes, the assessment focuses on specific machine learning models; other times, it focuses on a target organization’s overall data science maturity level. In addition, the underlying policies, procedures, and systems related to information security, data protection, and data privacy are also key drivers of future risk. AI companies stuffed full of data often make attractive targets for threat actors looking to profit from stolen data.
In practice, most investors or acquirers today simply rely on representations and warranties to shift the burden of this risk. But, as the FTC’s recent WW case demonstrates, reps and warranties will only get you so far. If your business scales or integrates the IP of an acquired company, the impact from penalties or disgorgement could significantly exceed the R&W caps in your purchase agreement.
Thankfully, these outcomes can be avoided by including data protection and machine learning model assessments in the technical diligence of potential investments and acquisitions. Think of it as a vaccine for your investment portfolio – a little prick now can prevent a lot of pain later.
For Board Members
As a board member, your role in overseeing AI compliance and mitigating regulatory risks like FTC enforcement actions is crucial. Here are some proactive measures to consider:
Establish an AI Ethics Committee: Create a dedicated committee or task an existing one with overseeing AI and data practices. This demonstrates a commitment to responsible AI use and provides a structured approach to addressing related risks.
Regular Risk Assessments: Insist on periodic assessments of the company’s AI and data practices. These should cover data acquisition methods, consent processes, and the potential for algorithmic bias or other ethical concerns.
Due Diligence in M&A: When considering acquisitions or investments, particularly in AI-driven companies, push for thorough due diligence on data practices and AI models. Be wary of potential “tainted” data or models that could lead to FTC actions.
Continuous Education: Stay informed about evolving AI regulations and FTC enforcement trends. Consider bringing in external experts to brief the board on emerging risks and best practices to address them.
Transparency and Disclosure: Advocate for clear, public-facing statements about the company’s AI and data practices. This transparency can build trust with stakeholders and demonstrate a commitment to ethical AI use.
Crisis Management Planning: Set a strategic vision for the best, but plan for the worst. Ensure that there’s a plan in place for responding to potential FTC investigations or enforcement actions; it should include communication strategies and immediate steps to address any identified issues.
By focusing on these areas, board members can play a proactive role in guiding their companies towards responsible AI practices, mitigating risks of FTC enforcement, and protecting long-term shareholder value.