· Announcements · 4 min read
Pioneering Responsible Data Science: A Framework for Ethical Innovation
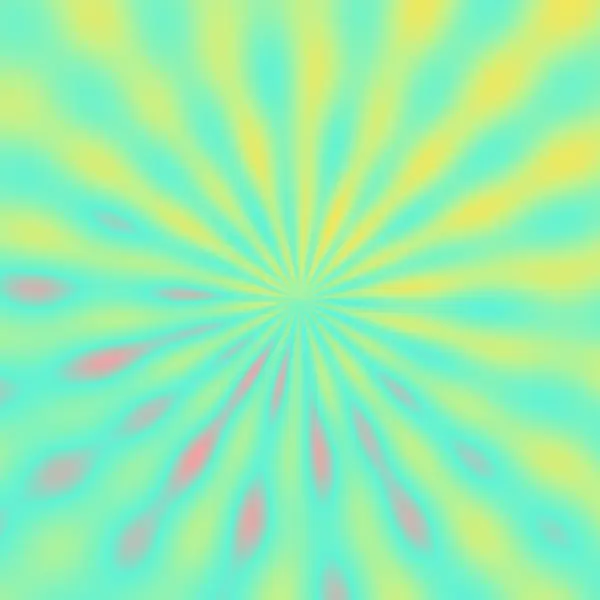
I created an open-source Responsible Data Science Policy Framework that addresses the urgent need for ethical AI governance in organizations, offering a flexible approach to risk management, legal compliance, and stakeholder trust.
Pioneering Responsible Data Science
In my role as a corporate advisor, I’ve witnessed firsthand the transformative power of data science and AI. All of the comic book fans out there know that with great power comes great responsibility; that’s why I’m excited to share my experience in helping create a Responsible Data Science Policy Framework through Licensio.
Why Now?
As discussed in the Licensio announcement post, less than half of the world’s largest organizations have governance procedures for AI ethics in place. This gap is particularly concerning given the increasing scrutiny from regulators and investors worldwide.
Regulators, especially in the US and EU, are drafting new rules for data science practices. Meanwhile, investors managing over $35 trillion in assets are incorporating data science-related ESG considerations into their funding criteria. The message is clear: responsible data science is no longer optional – it’s a necessity.
Bridging the Gap: Our Policy Framework
Recognizing this critical need, we have developed a Responsible Data Science Policy Framework. Our goal was to create a tool that could help organizations of all sizes navigate the complex landscape of data science compliance.
The framework is designed to address several key challenges:
- Risk Management: It helps organizations identify and mitigate risks associated with data science and AI applications.
- Legal Compliance: The framework considers emerging regulations, helping organizations stay ahead of legal requirements.
- Ethical Considerations: It incorporates ethical principles to ensure responsible use of data and AI technologies.
- Stakeholder Trust: By implementing this framework, organizations can build trust with customers, investors, and the public.
A Flexible and Evolving Approach
What sets this framework apart is its modular and adaptable design. It consists of:
- A parent procedure for triaging specific use cases or project types
- Prescriptive sub-procedures for low-friction compliance
- Adjudicative sub-procedures for centralized decision-making
This structure allows organizations to start with a basic committee-based approach and evolve towards more specialized, efficient processes over time. The result is a system that grows with your organization, continually improving clarity, reducing friction, and mitigating risks.
Why You Should Care
Strategic Oversight and Competitive Advantage: As data science becomes central to business strategy, having a robust governance framework sets you apart from competitors; board understanding of the ethical use of data science and AI is critical to maintaining this advantage in the long-term.
Risk Mitigation: Proactively addressing ethical and legal concerns can save your organization from costly mistakes and reputational damage. Given boards’ fiduciary responsibilities to oversee risk management, promoting a robust framework to manage the risks related to the ever-increasing use of data in traditional data science or AI model training helps boards fulfill this duty.
Innovation Enablement: A clear framework provides guardrails that actually enable faster, more confident innovation in data science. Boards must also ensure that such innovation occurs within ethical boundaries; a well-structured policy provides this balance, enabling responsible innovation.
Stakeholder Confidence: Demonstrating responsible practices builds trust with customers, investors, and regulators. Boards are increasingly expected to consider a broad range of stakeholders, and promoting a framework such as this one is demonstrable evidence that the board has considered a range of stakeholders.
Future-Proofing: As regulations evolve, having a flexible framework in place makes adaptation easier.
Though implementation of a responsible framework like this does not have to be initiated by the board, it’s often most effective to have “buy-in” at the top. By prioritizing the implementation of responsible data science policies, boards can ensure their organizations are not just compliant, but are leaders in ethical innovation. This proactive approach turns potential risks into strategic advantages, positioning the company for sustainable success in the data-driven future.
The Path Forward
Implementing a responsible data science policy isn’t just about compliance – it’s about building a better business and contributing to a better society. Whether you’re a startup just beginning to leverage AI or a large corporation with established data practices, this framework offers a roadmap to more ethical, efficient, and valuable data science operations.
As we navigate the exciting yet challenging world of AI and data science, frameworks like this will be essential. They help us harness the power of these technologies while ensuring we do so in a way that’s responsible, ethical, and beneficial to all stakeholders.
Open Source
I believe that by sharing my expertise in the area of responsible data science and ethical AI I can help organizations who would otherwise be unable to do what “should” be done do the right thing. We open sourced this framework to make it accessible to all organizations committed to responsible data practices.