· Connectors · 3 min read
The Critical Role of Data in AI Systems: Executive Summary
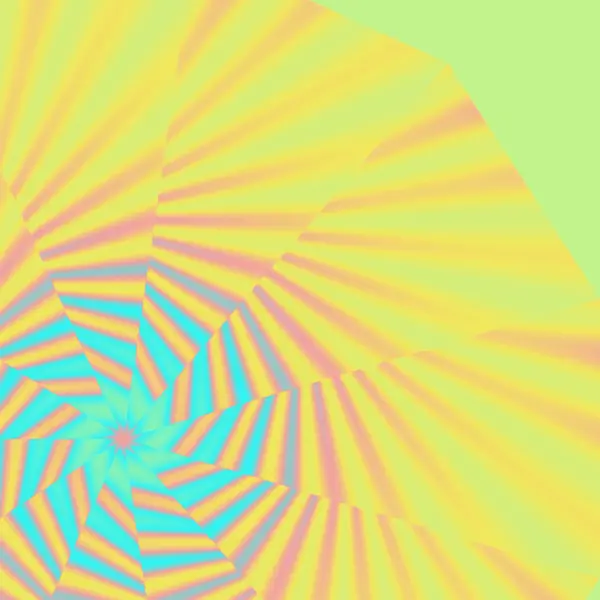
High-quality data is crucial for AI systems at every stage:
- Training: Foundational to model performance and capabilities
- Tuning: Essential for adapting models to specific domains or tasks
- Augmentation: Critical for enhancing model outputs and reducing errors
Board implications include data strategy oversight, risk management, and potential for competitive advantage through proprietary data assets.
The success and reliability of AI systems, particularly large language models (LLMs), are fundamentally dependent on the quality of data used throughout their lifecycle. Understanding this dependency is crucial for board members as organizations increasingly integrate AI into their operations and decision-making processes.
I wrote a thorough post over at 273 Ventures (my legal AI company) about why data matters in training, tuning and augmenting AI. If you’re interested in a deeper dive, you can explore that post; for this post I’ve distilled the information into the points that are most relevant to corporate boards.
Key Aspects of Data Importance in AI
Training: The Foundation of AI Models
- High-quality, diverse training data is essential for developing capable and reliable AI models
- Poor training data can lead to biased or underperforming models
- Many models share common data sources, potentially exposing multiple systems to similar risks (e.g., copyright issues)
Board Consideration: How can we ensure the integrity and diversity of our AI training data? What are the potential risks associated with our data sources?
Tuning: Adapting Models for Specific Uses
- Fine-tuning and delta-tuning allow organizations to customize general-purpose models for specific domains or tasks
- Quality domain-specific data is crucial for effective tuning
- Tuned models can provide significant competitive advantages in specialized applications
Board Consideration: How should we prioritize and resource the development of domain-specific datasets for AI tuning? What are the potential competitive advantages of custom-tuned AI models in our industry?
Augmentation: Enhancing AI Outputs
- Retrieval-augmented generation (RAG) combines AI models with external data sources to improve accuracy and reduce hallucinations
- High-quality, curated data is essential for effective RAG implementations
- Augmentation can significantly enhance the reliability and usefulness of AI outputs
Board Consideration: How can we leverage our existing organizational knowledge and data assets to enhance AI outputs? What investments in data curation and management are necessary to support effective AI augmentation?
Data Quality: A Cross-Cutting Concern
- The adage “garbage in, garbage out” applies strongly to AI systems
- Ensuring data quality is critical at all stages: training, tuning, and augmentation
- Poor data quality can lead to inaccurate outputs, biased decision-making, and potential regulatory or reputational risks
Board Consideration: How do we ensure and maintain data quality across our organization to support AI initiatives? What governance structures and processes need to be in place?
Strategic Implications for the Board
Data Strategy Oversight: The critical role of data in AI success necessitates a comprehensive organizational data strategy. How does our current data strategy align with our AI ambitions and overall business objectives?
Risk Management: Poor data quality or problematic data sources can introduce significant risks to AI systems. How are we identifying, assessing, and mitigating data-related risks in our AI initiatives?
Competitive Advantage: High-quality, proprietary datasets can provide significant competitive advantages in AI development and deployment. How are we leveraging our unique data assets to create value and differentiation through AI?
Investment Prioritization: Developing and maintaining high-quality datasets for AI requires significant resources. How should we prioritize investments in data acquisition, curation, and management to support our AI initiatives?
Ethical and Regulatory Compliance: The use of data in AI systems raises important ethical and regulatory considerations. How are we ensuring that our data practices for AI comply with relevant regulations and align with our ethical standards?
By recognizing the critical role of data in AI systems, boards can provide more effective oversight of AI initiatives, ensure appropriate resource allocation for data management, and guide their organizations toward responsible and value-creating AI adoption strategies.