· Explainer · 7 min read
AI Oversight: 5 Key Sources of Board Requirements
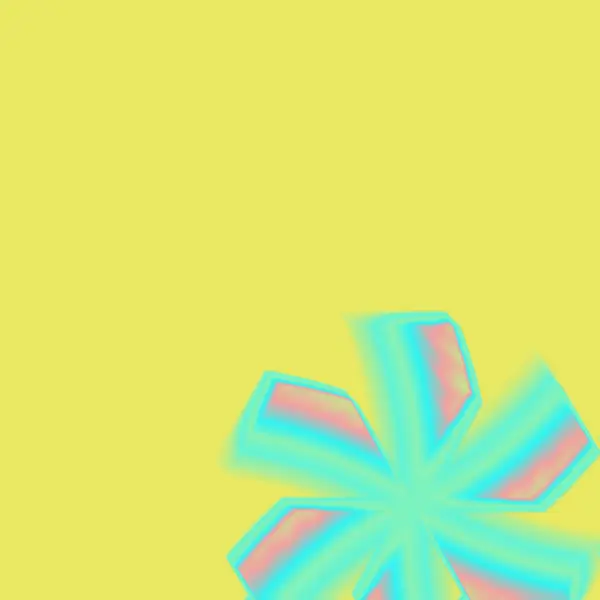
Boards’ understanding of the common requirements in AI procurement and deployment is essential for effective governance.
These requirements stem from five key sources:
- legal and regulatory mandates
- risk management frameworks
- insurance requirements
- internal policies
- customer/partner preferences
Common areas of concern include data governance and security, access control, operational resilience, human resources and third-party management, risk management and compliance, and emerging AI-specific considerations.
While procurement decisions are made well below the board level, the decision about if and how an organization will use AI falls within the strategic and governance oversight of the board.
This post covers common risk and compliance requirements that are shared enterprise-wide, regardless of the specific department or use case. My goal is to highlight typical requirements that arise during the procurement and deployment of AI solutions, but to frame them at a high-level that is relevant to corporate boards.
The Five Sources of Requirements
Corporate governance and oversight is driven by a number of “sources” - some of these may be internally-driven by the board, but others may be imposed upon the organization. This oversight in turn drives how organizations procure, deploy, and implement AI.
Requirements and constraints generally come from five different sources:
Legal and Regulatory Requirements: These are the most obvious source of constraints for organizations. In addition, given the sectoral nature of the US, many organizations are also subject to industry standards of professional “regulations” that impose obligations. Companies that operate globally often face complex compliance challenges that require careful planning and execution to manage effectively.
Risk Management Frameworks: These frameworks are another important source of obligations and constraints related to risk. They sometimes fall into the prior category, in the case that they have been incorporated into laws or regulations (e.g., National Institute of Standards and Technology (NIST) publications as a guide for minimum acceptable requirements). Other times, organizations may adopt them independently as a strategic risk management decision, as they are contractually obligated, or in response to changing industry practices.
Insurance Requirements: Essentially all mature organizations utilize external insurance coverage as a strategy for risk management. These policies often impose additional requirements on how technology can be used. For example, many professional liability or indemnity insurance providers have instituted additional transparency obligations that require policyholders to disclose how their organizations are using AI.
Even in cases where organizations are self-insured or have captive insurance companies, these requirements are often still present in the form of internal policies, as discussed below.
Internal Policies and Economics: Another significant source of constraints come from an organization’s internal policies. These policies may be driven by external forces, like those described above, or they may be based on the board and management’s preferences.
Economic considerations may constrain how organizations can realistically procure and deploy AI; boards serve a valuable role in managing the tension between the organization’s strategic vision, risk management, and financial operations.
Customer and Partner Preferences: Preferences of customers and partners can serve as another source of requirements or constraints for an organization. With respect to AI in particular, customers may request specific jurisdictional processing, which can limit which models or products an organization can utilize. Even when these preferences are not imposed by laws or regulations, your customer or partner’s internal procurement or risk management policies may require that you, the service or product providers, adhere to specific practices.
Common Requirements for AI
Regardless of the source of the requirements, organizations need to be prepared to comply with certain rules or standards when developing, procuring, and implementing AI. It’s an ongoing process to identify and manage these requirements, but there are some “common denominators” that must nearly always be addressed. I’ve outlined these common requirements into key categories below:
Data Governance and Security
Data at Rest: Rules concerning how and where data is stored, including jurisdictional requirements and encryption standards. For AI tools and solutions, these rules should apply wherever the data is at rest within the system, including all third-party databases.
Data in Transit: Requirements for how data is transmitted over networks, such as the use of private networks or specific encryption protocols. AI solutions frequently involve multiple API layers, so data flows (including the contents of inputs, outputs, and any attachments) should be mapped through all layers. This can be particularly difficult when the data crosses organizational boundaries (for example, when your company uses a vendor’s product that utilizes a third-party API for a model).
Data Processing: Rules about how data is processed, including requirements for single-tenant environments, dedicated servers, or explainable AI algorithms. These types of rules are often the most complex, yet most essential to ensuring that a given solution is compliant with data protection laws and regulations. These rules may not only cover the data that’s processed, but also the underlying algorithms that are used, as well as the software to run them.
Data Retention and Deletion: Policies governing how long data is kept and procedures for its deletion, including compliance with data subject requests. With AI solutions, this category is an example of “easier said than done.” The use of AI nearly always involves the flow of data across organizations. The more parties that are involved in the flow of data, the more difficult it can be to ensure that these policies are properly complied with. AI providers have realized the value of the data that they collect, so it’s essential that your company assesses whether the third parties’ data collection, use, and model training aligns with your organization’s data retention and deletion requirements.
Access Control and Monitoring
Authentication and Authorization: Rules for verifying user identities and controlling access to systems and data, such as multi-factor authentication requirements.
Audit and Reporting: Requirements for system audits and regular reporting on system and data status.
Operational Resilience
- Business Continuity and Disaster Recovery: Requirements for data backup and system restoration in case of disasters, including specific recovery time objectives. Assessing operational resilience related to AI yet again requires an understanding of the network of parties involved in processing and storing your data. Many AI-powered products rely on third-party LLM providers, like OpenAI, Anthropic, or Google; consequently, having a holistic view of business continuity requires not only understanding how your product vendor addresses it, but how the third-party model provider does as well.
Human Resources and Third-Party Management
Personnel Requirements: Rules concerning the hiring, training, and management of staff, including background checks and specific expertise requirements.
Third-Party Vendor Management: Policies for selecting, managing, and monitoring third-party vendors, including due diligence processes. Supply chain management for vendors is especially important for AI solutions, as they often integrate open source software and involve multiple service providers.
Risk Management and Compliance
Insurance and Risk Management: Requirements related to procuring and maintaining appropriate insurance coverage and other risk management practices.
Intellectual Property Rights and Data Ownership: AI is driven by data: policies for addressing the data ownership and intellectual property rights in the context of AI should establish requirements relating to the use of external AI products or systems and, to the extent applicable, to your internal development of AI.
Emerging AI-Specific Requirements
Given the nature of AI, additional requirements may emerge over time. Board directors may want to consider the following AI-specific requirements as they consider governance of this emerging technology:
AI Ethics and Fairness: Ensuring AI systems are designed and operate in an ethical manner, free from bias and discrimination.
AI Transparency and Explainability: Requirements for AI systems to provide clear explanations for their decisions or recommendations.
AI Safety and Robustness: Ensuring AI systems are safe, reliable, and perform consistently under various conditions.
AI Accountability: Establishing clear lines of responsibility and accountability for AI system outcomes.
Depending on the type of AI created or used by your organization (e.g., LLMs versus image classifiers), these requirements may not be easily achievable; it’s the board’s role, however, to ensure that the risks are managed in line with the organization’s risk management program.
Leveraging Common Requirements for Effective AI Oversight
By understanding the common requirements in AI use, board directors can better provide effective oversight of their organizations’ AI initiatives. Recognizing the five key sources of requirements - legal and regulatory, risk management frameworks, insurance, internal policies, and stakeholder expectations - provides boards with a comprehensive way to approach AI governance.
I’ve outlined these common requirements, from data governance and security to emerging AI-specific considerations, as a means by which boards can develop the framework they use to assess their organization’s readiness for AI adoption and ongoing use. These requirements should be considered more than compliance hurdles; they represent areas where boards can add value through strategic guidance and risk management.