· Explainer · 5 min read
Do You Know What Your Model Has Been Fed?
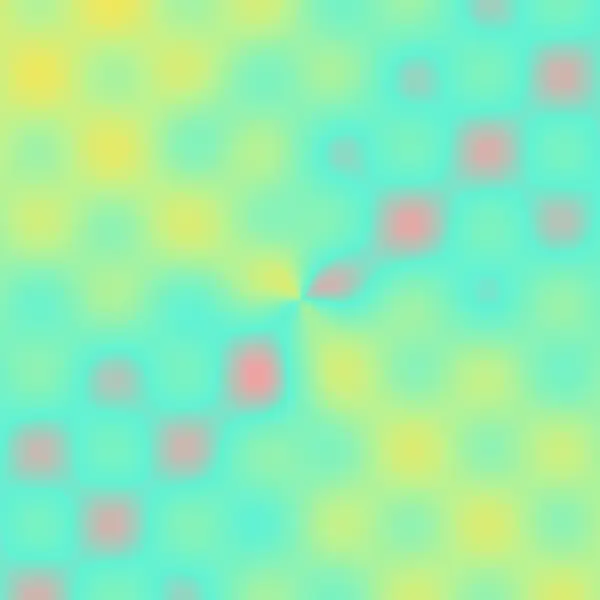
Pre-trained AI models offer great benefits but come with risks. Boards must implement robust governance frameworks to address data transparency, privacy, bias, compliance, and liability issues. This includes creating risk registers, conducting due diligence, establishing ethical guidelines, performing regular audits, and bringing in AI ethics expertise.
It’s hard to train a model from scratch. It’s expensive, time-consuming, and requires significant personnel resources (read: machine learning and AI experts cost $$$). Thankfully there are a number of pre-trained models available through platforms like Hugging Face; models like GPT-2 and T5 democratize access to immense AI capabilities.
It’s crucial, however, to understand the implications of these technologies, especially when it comes to pre-trained models and their training datasets (and even open source datasets more broadly). While these resources offer significant advantages, they also present unique challenges that demand careful consideration not only by the developers, but also by the board as they develop corporate governance related to AI.
Questions to Ask About Pre-trained Models
Though many of these questions apply regardless of whether an organization decides to train (or fine-tune) their own model or use a pre-trained model, certain considerations must be given greater weight based on the amount of control and insight that the organization has into the data.
Organizations must be prepared to ask themselves questions in the following areas:
Training Data Transparency: Do we have visibility into the data used to train these models? This question is fundamental, as it directly impacts the model’s output and potential biases. Without transparency, many of the questions below can’t be effectively answered.
Personal Information and Privacy: Was personal information included in the training data? If so, what are the implications for data protection regulations like GDPR or CCPA? There are usually many cascading questions in this category, but in some cases the answer is “no” and the organization can rest assured that this isn’t a risk.
Bias and Fairness: Are there inherent biases in the training data that could lead to unfair or discriminatory outcomes? How might this affect our products, services, or decision-making processes? Based on an organization’s use case(s), the exposure from this category of risk may be low or non-existent; for example, an embedding model that is used to classify a company’s clothing product SKUs into categories like pants or shirts has negligible risk associated with biases in the training data.
Licensing and Compliance: Have we thoroughly reviewed and understood the licensing terms for these models and datasets? Are our data scientists and developers using them in compliance with these terms? In some cases, these questions will need to be addressed by the organization’s legal team, but I’ve seen some pretty egregious oversights that should be caught by developers, like the commercial use of a model that is licensed explicitly for non-commercial use only (such as CC BY-NC 4.0).
Liability and Risk Management: In the event of issues arising from the use of open-source datasets or pre-trained models, what is our liability exposure? How does this change if we modify the models significantly? These kinds of questions don’t have clear legal precedent yet, so it’s important that the board establishes a clear risk framework that ensures that the organization can operate within the bounds of the organization’s risk tolerance with respect to the use of open source models or datasets.
Implementing a Robust Risk Assessment Framework
To address these concerns, boards should advocate for and oversee the implementation of a risk assessment framework as part of the organization’s comprehensive AI governance strategy:
Risk Register: Develop and maintain a detailed risk register that specifically addresses AI and ML model usage. This should be a living document, regularly updated to reflect new insights and emerging risks.
Due Diligence Process: Establish a rigorous due diligence process for vetting AI models and datasets before their integration into critical business processes.
Ethical AI Guidelines: Create and enforce clear guidelines for the ethical use of AI within the organization, addressing issues such as data privacy, fairness, and transparency. Good thing there are open source options available!
Regular Audits: Implement a schedule of regular audits to ensure ongoing compliance with licensing terms, data protection regulations, and internal ethical guidelines.
Expertise on the Board: Consider bringing AI and data ethics experts onto the board or forming an advisory committee to provide specialized guidance on these matters. This is one of my favorite types of engagements - please reach out to me if you’d like to discuss this.
Looking Ahead: The Future of AI Governance
As AI continues to advance, we may soon see more sophisticated tools for managing AI-related risks. Imagine a model that could review your company’s source code and generate the risk register by itself. While this prospect is exciting, it also raises the age-old question: “Quis custodiet ipsos custodes?” (Who will guard the guards themselves?)
This underscores the ongoing need for human oversight and judgment in AI governance. As board members and advisors, our role is to ensure that our organizations harness the power of AI responsibly, with a keen awareness of both its potential and its pitfalls.
By prioritizing these considerations, we can help steer our organizations toward a future where AI enhances business capabilities while upholding ethical standards and minimizing risks.
As the field of AI governance is rapidly evolving, engaging with consultants who have deep expertise in both AI technologies and corporate risk management can provide invaluable insights. These experts can help boards navigate the complex landscape of AI ethics, compliance, and risk mitigation strategies. If your organization is looking to enhance its AI governance capabilities, I offer advisory services in this area and would be happy to discuss how I can assist your board in developing robust AI risk management frameworks.